☞ AI, Semiotic Physics, and the Opcodes of Story World
Studying the humanities to understand language models
With the release of GPT-4, I’ve been exploring research related to the ideas of the pseudonymous janus, who is involved in the area of AI alignment. In particular, janus wrote an incredibly thoughtful essay laying out their take on the true nature of these large language models. After examining its particular properties, the essay eliminates these language models as being agents, oracles, tools, and even genies—these are technical terms!—before finally settling on simulators.
In other words, as per janus, these language models are designed to construct a model of the world, specifically based on predicting the next word in a prompt (‘all GPT “cares about” is simulating/modeling the training distribution’). These simulators can then generate specific kinds of simulacra, which are the “entities” simulated by the language models that we actually interact with.
But unlike simulations of the world based on physics or agent-based models (which I like to think about a lot), these simulators are models of a text world, or even a story world, not necessarily physical reality1 (there are also parallels here with my explorations last year with AI and alternate histories).
In fact, those involved in this have described it these kinds of AIs as having a semiotic physics, a term that I particularly love: “Semiotic physics is concerned with the dynamics of signs that are induced by simulators like GPT.”
The example that they begin with is coin flipping. In the real world, as you flip a coin more times, you approximate 50% heads and 50% tails, along with a certain specific distribution of the sequences of heads and tails in a row. But not so in the Story World of GPT. Sequences more often end in tails:
There are other features of Story World that are present. For example, the semiotic physics article even discusses the idea of Chekhov’s gun as a sort of “attractor” or primitive in the physics of GPT-like models.
Related to this, I’ve been thinking a lot about
’s idea of LLMs as a kind of “fuzzy processor.” But if these are processors or CPUs, what are the opcodes, the base instructions that we can use?Combining the metaphors of semiotic physics and chip architecture, perhaps the opcodes of LLMs are the tools of rhetoric and narrative structure.
In particularly, we must study these frameworks well, and figure out how to use them in language models:
One example where this has been done well is in elucidating the Waluigi effect (which also advocates for studying TV Tropes and the like), but there has been a broader call for imbibing this thinking about the world and story: “for prompt engineering, you'll need knowledge of literature, psychology, history, etc.”
In response, Hollis Robbins, Dean of the College of Humanities at the University of Utah has made this call even stronger:

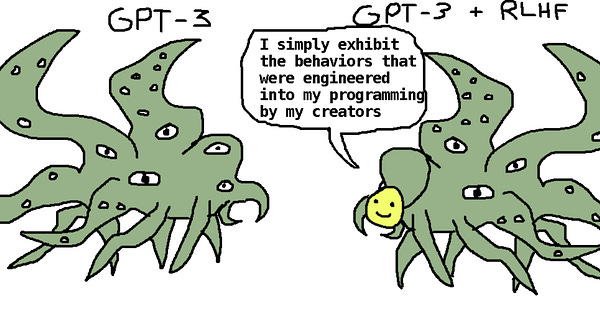
A better understanding of ideas and concepts from the humanities might allow us to study the following properties of these models’ Story Worlds:
Randomness
The nature of time
The nature of plot and story
The nature of perceived geography
Or they might even teach us about the Tower of Babel.
To give a flavor of this kind of approach, there is the idea, as per Wikipedia, of a “quibble”:
a quibble is a plot device, used to fulfill the exact verbal conditions of an agreement in order to avoid the intended meaning. Typically quibbles are used in legal bargains and, in fantasy, magically enforced ones.
While I’m still playing with this, I tried to use this idea of the quibble with ChatGPT, giving it the following prompt:
And I got an answer which began this way:
Not earth-shattering, but certainly intriguing.
So go study the concepts of narrative technique and use them to elucidate the behavior of LLMs. Or examine the rhetorical devices that writers and speakers have been using for millennia—and which GPT models has imbibed—and figure out how to use their “physical” principles in relating to these language models.
Ultimately, we need a deeper kind of cultural and humanistic competence, one that doesn’t just vaguely gesture at certain parts of history or specific literary styles. It’s still early days, but we need more of this thinking. To quote Hollis Robbins again: “Nobody yet knows what cultural competence will be in the AI era.” But we must begin to work this out.
It’s time to explore the Story World of AI. ■
Thanks to Hollis Robbins, , and for feedback on this essay.
The Enchanted Systems Roundup
Here are some links worth checking out that touch on the complex systems of our world (both built and natural):
🜑 AI-enhanced protein design makes proteins that have never existed: “One effective strategy to understand protein sequence and structure is to approach them as ‘text’, using language modeling algorithms that follow rules of biological ‘grammar’ and ‘syntax’.”
🜸 Upwelling: Combining real-time collaboration with version control for writers: “Instead of writers editing the document directly, a document is built out of layers, and every edit occurs within one. Layers are titled, have one or more authors, and support comments for discussion or review. A layer can be edited until it is merged and joins the stack. The stack contains the complete editing history of the document.”
🜹 The False Promise of Chomskyism: “I submit that, like the Jesuit astronomers declining to look through Galileo’s telescope, what Chomsky and his followers are ultimately angry at is reality itself, for having the temerity to offer something up that they didn’t predict and that doesn’t fit their worldview.”
🝳 AI and Image Generation (Everything is a Remix Part 4): Video that examines creativity in the age AI.
🜛 Silicon or Carbon? “If the previous era of tech was about bringing our physical world online, the decades to come will be defined by the race to open the next frontier for humanity.”
🝯 The Forgotten Story of Modulex: LEGO's Lost Cousin: Fascinating video.
🜑 Babel: Or the Necessity of Violence: An Arcane History of the Oxford Translators' Revolution by R.F. Kuang: Translation, magic, industry, empire, and more.
🝖 Hidden Systems: Water, Electricity, the Internet, and the Secrets Behind the Systems We Use Every Day by Dan Nott: “writer and cartoonist Dan Nott sheds light on three critical systems we so take for granted we barely see them even though they are all around us and often in plain sight.”
🜚 Kottke.org Is 25 Years Old Today and I’m Going to Write About It: I’ve been reading it for around 20 years.
🝤 Vesuvius Challenge: “Resurrect an ancient library from the ashes of a volcano.
Win $250,000. The Vesuvius Challenge is a machine learning and computer vision competition to read the Herculaneum Papyri.”
🝊 Anyone else witnessing a panic inside NLP orgs of big tech companies?
Until next time.
Because there is a stochastic nature to these systems, janus has even written of this in terms of exploring the multiverse that these language models generate:
A multiversal measure of impulse response is taken by perturbing something about the prompt - say, switching a character’s gender pronouns, or injecting a hint about a puzzle - and then comparing the sampled downstream multiverses of the perturbed and unperturbed prompts. How this comparison is to be done is, again, an infinitely open question."
This is also related to the oft-mentioned idea that storytelling and fiction are ways for the human imagination to experience a large number of simulated lives, or futures, or diverse experiences, which of course we cannot do in reality (also discussed by janus).
And compare this to what
has noted: ‘AI might be usefully reframed as “the study of intelligence as it could be.”’
I really like this post, thank you. I wish I had more time to think about this stuff, but one thought I had recently, which I think fits together with what you have written here, is to think about the context window for LLMs. I think that sets the boundary of the length of any specific grammatical structure or simulation. Maybe, and this is where I’m speculating, but maybe if we think about language as emergent from our interaction with the world, we might be able to think about these LLMS as containing huge sets of cassettes of functional grammatical mini programs. Sone of these operate well in the world, and some have less direct applicability, but it might give a hint as to why these things are so damn useful.
For dedicated story creation, Dramatron will be more interesting to study, and its latest test run has been analyzed here: https://deepai.org/publication/co-writing-screenplays-and-theatre-scripts-with-language-models-an-evaluation-by-industry-professionals
ChatGPT is easier to use for now, but still, it needs to be trained, and the amount of time I spent on it so far never yielded more than basic, generic output. It will improve over time, no doubt.